Data Science vs Data Analytics – Difference between Data Science and Data Analytics
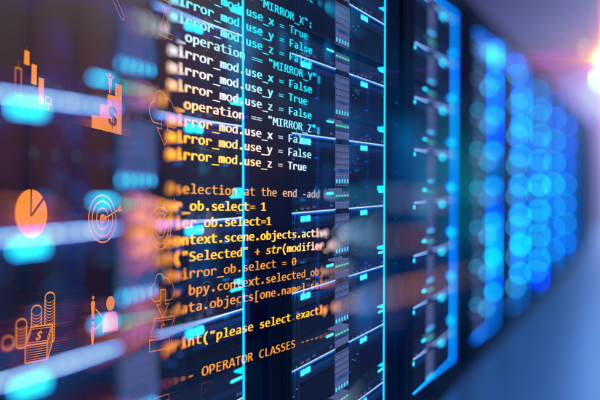
When it comes to the world of data, there are two terms that often get used interchangeably: data science and data analytics. While they may sound similar, there are key differences between the two that are worth exploring.
Data science is a multidisciplinary field that combines scientific methods, algorithms, and systems to extract knowledge and insights from structured and unstructured data. It involves various techniques like machine learning, statistical analysis, and data visualization to uncover patterns, make predictions, and solve complex problems.
On the other hand, data analytics focuses more on the process of examining, cleaning, transforming, and modeling data to discover useful information, draw conclusions, and support decision-making. It involves descriptive and diagnostic analysis to understand what happened and why it happened.
While data science and data analytics share some similarities, the main distinction lies in their objectives and scope. Data science aims to generate actionable insights and build predictive models, while data analytics focuses on understanding and interpreting data to drive business decisions.
In terms of skills required, data scientists typically have a strong background in mathematics, statistics, and programming. They are proficient in coding languages like Python or R and have a deep understanding of machine learning algorithms. Data analysts, on the other hand, focus more on data manipulation, visualization, and reporting. They need to be skilled in tools like SQL and Excel.
Both data science and data analytics play crucial roles in today’s data-driven world. Organizations can leverage the power of data to gain a competitive advantage, improve customer experiences, and drive innovation. By understanding the differences between the two, businesses can make informed decisions about which approach is best suited for their specific needs.
Defining Data Science
Data Science is a multidisciplinary field that combines elements of mathematics, statistics, computer science, and domain-specific knowledge to uncover hidden patterns, extract valuable insights, and build predictive models. It’s a holistic approach to data, often referred to as the “sexiest job of the 21st century” by Harvard Business Review. Data scientists are akin to modern-day detectives, tasked with exploring complex data sets to solve problems and extract actionable knowledge.
Key characteristics of Data Science include:
Data Exploration: Data scientists spend a significant amount of time exploring and cleaning data. This is essential because real-world data is often messy, incomplete, and unstructured.
Machine Learning: Machine learning, a subset of artificial intelligence, is a crucial component of data science. Data scientists use algorithms to create predictive models and make data-driven decisions.
Predictive Analytics: Data science involves predictive modeling to forecast future trends and events, such as sales forecasts, customer churn predictions, and demand forecasting.
Big Data: Data scientists often work with massive data sets, making use of technologies like Hadoop and Spark to handle and analyze data at scale.
Deep Learning: Deep learning, a subset of machine learning, is utilized in data science for tasks like image recognition, natural language processing, and recommendation systems.
Defining Data Analytics
Data Analytics, on the other hand, is the process of examining, cleaning, transforming, and visualizing data to uncover valuable insights and support decision-making. It is more focused on providing answers to specific questions or solving immediate problems by summarizing and interpreting data.
Key characteristics of Data Analytics include:
Descriptive Analytics: Data analytics is primarily concerned with what happened in the past. It involves creating reports, dashboards, and visualizations to describe historical data trends.
Business Intelligence: Data analysts often work closely with business stakeholders to understand their needs and deliver actionable insights that can inform day-to-day decisions.
Statistical Analysis: Data analysts utilize statistical techniques to draw meaningful conclusions from data. These techniques may include regression analysis, hypothesis testing, and A/B testing.
Data Visualization: Data analytics heavily involves creating charts, graphs, and dashboards to make data more accessible and understandable for non-technical stakeholders.
Key Differences Between Data Science and Data Analytics
Scope and Purpose: Data science has a broader scope, focusing on predictive modeling, machine learning, and big data technologies. Data analytics, on the other hand, has a narrower focus on providing descriptive insights and supporting immediate business decisions.
Time Perspective: Data science is more future-oriented, as it seeks to predict future trends and events. Data analytics is past-oriented, emphasizing what has already happened.
Skills Required: Data scientists need strong programming, statistical, and machine learning skills. Data analysts require strong data visualization and business intelligence skills.
Tools and Technologies: Data scientists work with big data technologies, complex algorithms, and deep learning frameworks. Data analysts use tools like Excel, Tableau, and Power BI for data visualization and reporting.
End Goal: Data scientists often build complex models that can be integrated into production systems, while data analysts focus on providing insights to support day-to-day operations and decision-making.
Conclusion
In the world of data, both Data Science and Data Analytics play vital roles, but they serve different purposes and require distinct skill sets. Data science is the broader field, focused on using advanced techniques to predict future trends and make complex, data-driven decisions. Data analytics, on the other hand, is more focused on providing insights about past events and supporting immediate business decisions.
Understanding the differences between these two fields is essential for organizations seeking to harness the power of data effectively. Depending on your specific goals, you can choose whether to invest in data science, data analytics, or a combination of both to extract the most value from your data assets. Ultimately, it’s about finding the right balance between data exploration, analysis, and modeling to achieve your business objectives.